Many machine learning models are capable of predicting a probability or probability-like scores for class membership.
Probabilities provide a required level of granularity for evaluating and comparing models, especially on imbalanced classification problems where tools like ROC Curves are used to interpret predictions and the ROC AUC metric is used to compare model performance, both of which use probabilities.
Unfortunately, the probabilities or probability-like scores predicted by many models are not calibrated. This means that they may be over-confident in some cases and under-confident in other cases. Worse still, the severely skewed class distribution present in imbalanced classification tasks may result in even more bias in the predicted probabilities as they over-favor predicting the majority class.
As such, it is often a good idea to calibrate the predicted probabilities for nonlinear machine learning models prior to evaluating their performance. Further, it is good practice to calibrate probabilities in general when working with imbalanced datasets, even of models like logistic regression that predict well-calibrated probabilities when the class labels are balanced.
In this tutorial, you will discover how to calibrate predicted probabilities for imbalanced classification.
After completing this tutorial, you will know:
- Calibrated probabilities are required to get the most out of models for imbalanced classification problems.
- How to calibrate predicted probabilities for nonlinear models like SVMs, decision trees, and KNN.
- How to grid search different probability calibration methods on a dataset with a skewed class distribution.
Kick-start your project with my new book Imbalanced Classification with Python, including step-by-step tutorials and the Python source code files for all examples.
Let’s get started.
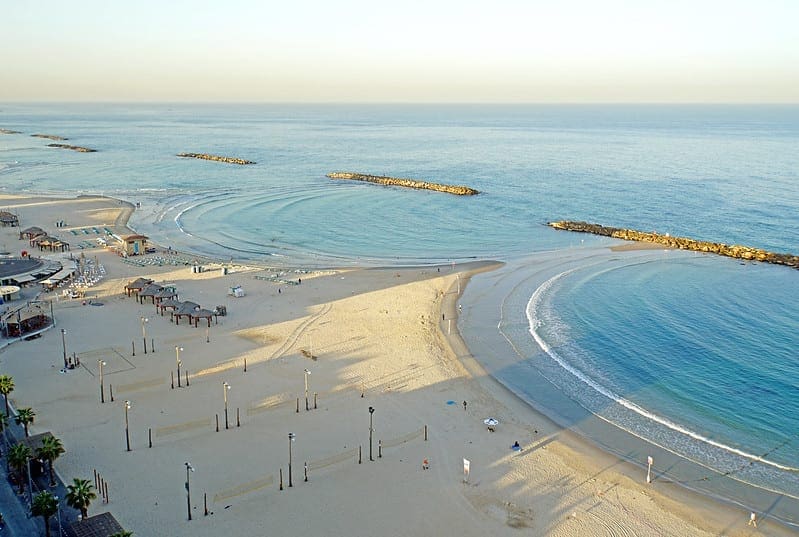
How to Calibrate Probabilities for Imbalanced Classification
Photo by Dennis Jarvis, some rights reserved.
Tutorial Overview
This tutorial is divided into five parts; they are:
- Problem of Uncalibrated Probabilities
- How to Calibrate Probabilities
- SVM With Calibrated Probabilities
- Decision Tree With Calibrated Probabilities
- Grid Search Probability Calibration with KNN
Problem of Uncalibrated Probabilities
Many machine learning algorithms can predict a probability or a probability-like score that indicates class membership.
For example, logistic regression can predict the probability of class membership directly and support vector machines can predict a score that is not a probability but could be interpreted as a probability.
The probability can be used as a measure of uncertainty on those problems where a probabilistic prediction is required. This is particularly the case in imbalanced classification, where crisp class labels are often insufficient both in terms of evaluating and selecting a model. The predicted probability provides the basis for more granular model evaluation and selection, such as through the use of ROC and Precision-Recall diagnostic plots, metrics like ROC AUC, and techniques like threshold moving.
As such, using machine learning models that predict probabilities is generally preferred when working on imbalanced classification tasks. The problem is that few machine learning models have calibrated probabilities.
… to be usefully interpreted as probabilities, the scores should be calibrated.
— Page 57, Learning from Imbalanced Data Sets, 2018.
Calibrated probabilities means that the probability reflects the likelihood of true events.
This might be confusing if you consider that in classification, we have class labels that are correct or not instead of probabilities. To clarify, recall that in binary classification, we are predicting a negative or positive case as class 0 or 1. If 100 examples are predicted with a probability of 0.8, then 80 percent of the examples will have class 1 and 20 percent will have class 0, if the probabilities are calibrated. Here, calibration is the concordance of predicted probabilities with the occurrence of positive cases.
Uncalibrated probabilities suggest that there is a bias in the probability scores, meaning the probabilities are overconfident or under-confident in some cases.
- Calibrated Probabilities. Probabilities match the true likelihood of events.
- Uncalibrated Probabilities. Probabilities are over-confident and/or under-confident.
This is common for machine learning models that are not trained using a probabilistic framework and for training data that has a skewed distribution, like imbalanced classification tasks.
There are two main causes for uncalibrated probabilities; they are:
- Algorithms not trained using a probabilistic framework.
- Biases in the training data.
Few machine learning algorithms produce calibrated probabilities. This is because for a model to predict calibrated probabilities, it must explicitly be trained under a probabilistic framework, such as maximum likelihood estimation. Some examples of algorithms that provide calibrated probabilities include:
- Logistic Regression.
- Linear Discriminant Analysis.
- Naive Bayes.
- Artificial Neural Networks.
Many algorithms either predict a probability-like score or a class label and must be coerced in order to produce a probability-like score. As such, these algorithms often require their “probabilities” to be calibrated prior to use. Examples include:
- Support Vector Machines.
- Decision Trees.
- Ensembles of Decision Trees (bagging, random forest, gradient boosting).
- k-Nearest Neighbors.
A bias in the training dataset, such as a skew in the class distribution, means that the model will naturally predict a higher probability for the majority class than the minority class on average.
The problem is, models may overcompensate and give too much focus to the majority class. This even applies to models that typically produce calibrated probabilities like logistic regression.
… class probability estimates attained via supervised learning in imbalanced scenarios systematically underestimate the probabilities for minority class instances, despite ostensibly good overall calibration.
— Class Probability Estimates are Unreliable for Imbalanced Data (and How to Fix Them), 2012.
Want to Get Started With Imbalance Classification?
Take my free 7-day email crash course now (with sample code).
Click to sign-up and also get a free PDF Ebook version of the course.
How to Calibrate Probabilities
Probabilities are calibrated by rescaling their values so they better match the distribution observed in the training data.
… we desire that the estimated class probabilities are reflective of the true underlying probability of the sample. That is, the predicted class probability (or probability-like value) needs to be well-calibrated. To be well-calibrated, the probabilities must effectively reflect the true likelihood of the event of interest.
— Page 249, Applied Predictive Modeling, 2013.
Probability predictions are made on training data and the distribution of probabilities is compared to the expected probabilities and adjusted to provide a better match. This often involves splitting a training dataset and using one portion to train the model and another portion as a validation set to scale the probabilities.
There are two main techniques for scaling predicted probabilities; they are Platt scaling and isotonic regression.
- Platt Scaling. Logistic regression model to transform probabilities.
- Isotonic Regression. Weighted least-squares regression model to transform probabilities.
Platt scaling is a simpler method and was developed to scale the output from a support vector machine to probability values. It involves learning a logistic regression model to perform the transform of scores to calibrated probabilities. Isotonic regression is a more complex weighted least squares regression model. It requires more training data, although it is also more powerful and more general. Here, isotonic simply refers to monotonically increasing mapping of the original probabilities to the rescaled values.
Platt Scaling is most effective when the distortion in the predicted probabilities is sigmoid-shaped. Isotonic Regression is a more powerful calibration method that can correct any monotonic distortion.
— Predicting Good Probabilities With Supervised Learning, 2005.
The scikit-learn library provides access to both Platt scaling and isotonic regression methods for calibrating probabilities via the CalibratedClassifierCV class.
This is a wrapper for a model (like an SVM). The preferred scaling technique is defined via the “method” argument, which can be ‘sigmoid‘ (Platt scaling) or ‘isotonic‘ (isotonic regression).
Cross-validation is used to scale the predicted probabilities from the model, set via the “cv” argument. This means that the model is fit on the training set and calibrated on the test set, and this process is repeated k-times for the k-folds where predicted probabilities are averaged across the runs.
Setting the “cv” argument depends on the amount of data available, although values such as 3 or 5 can be used. Importantly, the split is stratified, which is important when using probability calibration on imbalanced datasets that often have very few examples of the positive class.
1 2 3 4 |
... # example of wrapping a model with probability calibration model = ... calibrated = CalibratedClassifierCV(model, method='sigmoid', cv=3) |
Now that we know how to calibrate probabilities, let’s look at some examples of calibrating probability for models on an imbalanced classification dataset.
SVM With Calibrated Probabilities
In this section, we will review how to calibrate the probabilities for an SVM model on an imbalanced classification dataset.
First, let’s define a dataset using the make_classification() function. We will generate 10,000 examples, 99 percent of which will belong to the negative case (class 0) and 1 percent will belong to the positive case (class 1).
1 2 3 4 |
... # generate dataset X, y = make_classification(n_samples=10000, n_features=2, n_redundant=0, n_clusters_per_class=1, weights=[0.99], flip_y=0, random_state=4) |
Next, we can define an SVM with default hyperparameters. This means that the model is not tuned to the dataset, but will provide a consistent basis of comparison.
1 2 3 |
... # define model model = SVC(gamma='scale') |
We can then evaluate this model on the dataset using repeated stratified k-fold cross-validation with three repeats of 10-folds.
We will evaluate the model using ROC AUC and calculate the mean score across all repeats and folds. The ROC AUC will make use of the uncalibrated probability-like scores provided by the SVM.
1 2 3 4 5 6 7 |
... # define evaluation procedure cv = RepeatedStratifiedKFold(n_splits=10, n_repeats=3, random_state=1) # evaluate model scores = cross_val_score(model, X, y, scoring='roc_auc', cv=cv, n_jobs=-1) # summarize performance print('Mean ROC AUC: %.3f' % mean(scores)) |
Tying this together, the complete example is listed below.
1 2 3 4 5 6 7 8 9 10 11 12 13 14 15 16 17 |
# evaluate svm with uncalibrated probabilities for imbalanced classification from numpy import mean from sklearn.datasets import make_classification from sklearn.model_selection import cross_val_score from sklearn.model_selection import RepeatedStratifiedKFold from sklearn.svm import SVC # generate dataset X, y = make_classification(n_samples=10000, n_features=2, n_redundant=0, n_clusters_per_class=1, weights=[0.99], flip_y=0, random_state=4) # define model model = SVC(gamma='scale') # define evaluation procedure cv = RepeatedStratifiedKFold(n_splits=10, n_repeats=3, random_state=1) # evaluate model scores = cross_val_score(model, X, y, scoring='roc_auc', cv=cv, n_jobs=-1) # summarize performance print('Mean ROC AUC: %.3f' % mean(scores)) |
Running the example evaluates the SVM with uncalibrated probabilities on the imbalanced classification dataset.
Note: Your results may vary given the stochastic nature of the algorithm or evaluation procedure, or differences in numerical precision. Consider running the example a few times and compare the average outcome.
In this case, we can see that the SVM achieved a ROC AUC of about 0.804.
1 |
Mean ROC AUC: 0.804 |
Next, we can try using the CalibratedClassifierCV class to wrap the SVM model and predict calibrated probabilities.
We are using stratified 10-fold cross-validation to evaluate the model; that means 9,000 examples are used for train and 1,000 for test on each fold.
With CalibratedClassifierCV and 3-folds, the 9,000 examples of one fold will be split into 6,000 for training the model and 3,000 for calibrating the probabilities. This does not leave many examples of the minority class, e.g. 90/10 in 10-fold cross-validation, then 60/30 for calibration.
When using calibration, it is important to work through these numbers based on your chosen model evaluation scheme and either adjust the number of folds to ensure the datasets are sufficiently large or even switch to a simpler train/test split instead of cross-validation if needed. Experimentation might be required.
We will define the SVM model as before, then define the CalibratedClassifierCV with isotonic regression, then evaluate the calibrated model via repeated stratified k-fold cross-validation.
1 2 3 4 5 |
... # define model model = SVC(gamma='scale') # wrap the model calibrated = CalibratedClassifierCV(model, method='isotonic', cv=3) |
Because SVM probabilities are not calibrated by default, we would expect that calibrating them would result in an improvement to the ROC AUC that explicitly evaluates a model based on their probabilities.
Tying this together, the full example below of evaluating SVM with calibrated probabilities is listed below.
1 2 3 4 5 6 7 8 9 10 11 12 13 14 15 16 17 18 19 20 |
# evaluate svm with calibrated probabilities for imbalanced classification from numpy import mean from sklearn.datasets import make_classification from sklearn.model_selection import cross_val_score from sklearn.model_selection import RepeatedStratifiedKFold from sklearn.calibration import CalibratedClassifierCV from sklearn.svm import SVC # generate dataset X, y = make_classification(n_samples=10000, n_features=2, n_redundant=0, n_clusters_per_class=1, weights=[0.99], flip_y=0, random_state=4) # define model model = SVC(gamma='scale') # wrap the model calibrated = CalibratedClassifierCV(model, method='isotonic', cv=3) # define evaluation procedure cv = RepeatedStratifiedKFold(n_splits=10, n_repeats=3, random_state=1) # evaluate model scores = cross_val_score(calibrated, X, y, scoring='roc_auc', cv=cv, n_jobs=-1) # summarize performance print('Mean ROC AUC: %.3f' % mean(scores)) |
Running the example evaluates the SVM with calibrated probabilities on the imbalanced classification dataset.
Note: Your results may vary given the stochastic nature of the algorithm or evaluation procedure, or differences in numerical precision. Consider running the example a few times and compare the average outcome.
In this case, we can see that the SVM achieved a lift in ROC AUC from about 0.804 to about 0.875.
1 |
Mean ROC AUC: 0.875 |
Probability calibration can be evaluated in conjunction with other modifications to the algorithm or dataset to address the skewed class distribution.
For example, SVM provides the “class_weight” argument that can be set to “balanced” to adjust the margin to favor the minority class. We can include this change to SVM and calibrate the probabilities, and we might expect to see a further lift in model skill; for example:
1 2 3 |
... # define model model = SVC(gamma='scale', class_weight='balanced') |
Tying this together, the complete example of a class weighted SVM with calibrated probabilities is listed below.
1 2 3 4 5 6 7 8 9 10 11 12 13 14 15 16 17 18 19 20 |
# evaluate weighted svm with calibrated probabilities for imbalanced classification from numpy import mean from sklearn.datasets import make_classification from sklearn.model_selection import cross_val_score from sklearn.model_selection import RepeatedStratifiedKFold from sklearn.calibration import CalibratedClassifierCV from sklearn.svm import SVC # generate dataset X, y = make_classification(n_samples=10000, n_features=2, n_redundant=0, n_clusters_per_class=1, weights=[0.99], flip_y=0, random_state=4) # define model model = SVC(gamma='scale', class_weight='balanced') # wrap the model calibrated = CalibratedClassifierCV(model, method='isotonic', cv=3) # define evaluation procedure cv = RepeatedStratifiedKFold(n_splits=10, n_repeats=3, random_state=1) # evaluate model scores = cross_val_score(calibrated, X, y, scoring='roc_auc', cv=cv, n_jobs=-1) # summarize performance print('Mean ROC AUC: %.3f' % mean(scores)) |
Running the example evaluates the class-weighted SVM with calibrated probabilities on the imbalanced classification dataset.
Note: Your results may vary given the stochastic nature of the algorithm or evaluation procedure, or differences in numerical precision. Consider running the example a few times and compare the average outcome.
In this case, we can see that the SVM achieved a further lift in ROC AUC from about 0.875 to about 0.966.
1 |
Mean ROC AUC: 0.966 |
Decision Tree With Calibrated Probabilities
Decision trees are another highly effective machine learning that does not naturally produce probabilities.
Instead, class labels are predicted directly and a probability-like score can be estimated based on the distribution of examples in the training dataset that fall into the leaf of the tree that is predicted for the new example. As such, the probability scores from a decision tree should be calibrated prior to being evaluated and used to select a model.
We can define a decision tree using the DecisionTreeClassifier scikit-learn class.
The model can be evaluated with uncalibrated probabilities on our synthetic imbalanced classification dataset.
The complete example is listed below.
1 2 3 4 5 6 7 8 9 10 11 12 13 14 15 16 17 |
# evaluate decision tree with uncalibrated probabilities for imbalanced classification from numpy import mean from sklearn.datasets import make_classification from sklearn.model_selection import cross_val_score from sklearn.model_selection import RepeatedStratifiedKFold from sklearn.tree import DecisionTreeClassifier # generate dataset X, y = make_classification(n_samples=10000, n_features=2, n_redundant=0, n_clusters_per_class=1, weights=[0.99], flip_y=0, random_state=4) # define model model = DecisionTreeClassifier() # define evaluation procedure cv = RepeatedStratifiedKFold(n_splits=10, n_repeats=3, random_state=1) # evaluate model scores = cross_val_score(model, X, y, scoring='roc_auc', cv=cv, n_jobs=-1) # summarize performance print('Mean ROC AUC: %.3f' % mean(scores)) |
Running the example evaluates the decision tree with uncalibrated probabilities on the imbalanced classification dataset.
Note: Your results may vary given the stochastic nature of the algorithm or evaluation procedure, or differences in numerical precision. Consider running the example a few times and compare the average outcome.
In this case, we can see that the decision tree achieved a ROC AUC of about 0.842.
1 |
Mean ROC AUC: 0.842 |
We can then evaluate the same model using the calibration wrapper.
In this case, we will use the Platt Scaling method configured by setting the “method” argument to “sigmoid“.
1 2 3 |
... # wrap the model calibrated = CalibratedClassifierCV(model, method='sigmoid', cv=3) |
The complete example of evaluating the decision tree with calibrated probabilities for imbalanced classification is listed below.
1 2 3 4 5 6 7 8 9 10 11 12 13 14 15 16 17 18 19 20 |
# decision tree with calibrated probabilities for imbalanced classification from numpy import mean from sklearn.datasets import make_classification from sklearn.model_selection import cross_val_score from sklearn.model_selection import RepeatedStratifiedKFold from sklearn.calibration import CalibratedClassifierCV from sklearn.tree import DecisionTreeClassifier # generate dataset X, y = make_classification(n_samples=10000, n_features=2, n_redundant=0, n_clusters_per_class=1, weights=[0.99], flip_y=0, random_state=4) # define model model = DecisionTreeClassifier() # wrap the model calibrated = CalibratedClassifierCV(model, method='sigmoid', cv=3) # define evaluation procedure cv = RepeatedStratifiedKFold(n_splits=10, n_repeats=3, random_state=1) # evaluate model scores = cross_val_score(calibrated, X, y, scoring='roc_auc', cv=cv, n_jobs=-1) # summarize performance print('Mean ROC AUC: %.3f' % mean(scores)) |
Running the example evaluates the decision tree with calibrated probabilities on the imbalanced classification dataset.
Note: Your results may vary given the stochastic nature of the algorithm or evaluation procedure, or differences in numerical precision. Consider running the example a few times and compare the average outcome.
In this case, we can see that the decision tree achieved a lift in ROC AUC from about 0.842 to about 0.859.
1 |
Mean ROC AUC: 0.859 |
Grid Search Probability Calibration With KNN
Probability calibration can be sensitive to both the method and the way in which the method is employed.
As such, it is a good idea to test a suite of different probability calibration methods on your model in order to discover what works best for your dataset. One approach is to treat the calibration method and cross-validation folds as hyperparameters and tune them. In this section, we will look at using a grid search to tune these hyperparameters.
The k-nearest neighbor, or KNN, algorithm is another nonlinear machine learning algorithm that predicts a class label directly and must be modified to produce a probability-like score. This often involves using the distribution of class labels in the neighborhood.
We can evaluate a KNN with uncalibrated probabilities on our synthetic imbalanced classification dataset using the KNeighborsClassifier class with a default neighborhood size of 5.
The complete example is listed below.
1 2 3 4 5 6 7 8 9 10 11 12 13 14 15 16 17 |
# evaluate knn with uncalibrated probabilities for imbalanced classification from numpy import mean from sklearn.datasets import make_classification from sklearn.model_selection import cross_val_score from sklearn.model_selection import RepeatedStratifiedKFold from sklearn.neighbors import KNeighborsClassifier # generate dataset X, y = make_classification(n_samples=10000, n_features=2, n_redundant=0, n_clusters_per_class=1, weights=[0.99], flip_y=0, random_state=4) # define model model = KNeighborsClassifier() # define evaluation procedure cv = RepeatedStratifiedKFold(n_splits=10, n_repeats=3, random_state=1) # evaluate model scores = cross_val_score(model, X, y, scoring='roc_auc', cv=cv, n_jobs=-1) # summarize performance print('Mean ROC AUC: %.3f' % mean(scores)) |
Running the example evaluates the KNN with uncalibrated probabilities on the imbalanced classification dataset.
Note: Your results may vary given the stochastic nature of the algorithm or evaluation procedure, or differences in numerical precision. Consider running the example a few times and compare the average outcome.
In this case, we can see that the KNN achieved a ROC AUC of about 0.864.
1 |
Mean ROC AUC: 0.864 |
Knowing that the probabilities are dependent on the neighborhood size and are uncalibrated, we would expect that some calibration would improve the performance of the model using ROC AUC.
Rather than spot-checking one configuration of the CalibratedClassifierCV class, we will instead use the GridSearchCV to grid search different configurations.
First, the model and calibration wrapper are defined as before.
1 2 3 4 5 |
... # define model model = KNeighborsClassifier() # wrap the model calibrated = CalibratedClassifierCV(model) |
We will test both “sigmoid” and “isotonic” “method” values, and different “cv” values in [2,3,4]. Recall that “cv” controls the split of the training dataset that is used to estimate the calibrated probabilities.
We can define the grid of parameters as a dict with the names of the arguments to the CalibratedClassifierCV we want to tune and provide lists of values to try. This will test 3 * 2 or 6 different combinations.
1 2 3 |
... # define grid param_grid = dict(cv=[2,3,4], method=['sigmoid','isotonic']) |
We can then define the GridSearchCV with the model and grid of parameters and use the same repeated stratified k-fold cross-validation we used before to evaluate each parameter combination.
1 2 3 4 5 6 7 |
... # define evaluation procedure cv = RepeatedStratifiedKFold(n_splits=10, n_repeats=3, random_state=1) # define grid search grid = GridSearchCV(estimator=calibrated, param_grid=param_grid, n_jobs=-1, cv=cv, scoring='roc_auc') # execute the grid search grid_result = grid.fit(X, y) |
Once evaluated, we will then summarize the configuration found with the highest ROC AUC, then list the results for all combinations.
1 2 3 4 5 6 7 8 |
# report the best configuration print("Best: %f using %s" % (grid_result.best_score_, grid_result.best_params_)) # report all configurations means = grid_result.cv_results_['mean_test_score'] stds = grid_result.cv_results_['std_test_score'] params = grid_result.cv_results_['params'] for mean, stdev, param in zip(means, stds, params): print("%f (%f) with: %r" % (mean, stdev, param)) |
Tying this together, the complete example of grid searching probability calibration for imbalanced classification with a KNN model is listed below.
1 2 3 4 5 6 7 8 9 10 11 12 13 14 15 16 17 18 19 20 21 22 23 24 25 26 27 28 29 30 |
# grid search probability calibration with knn for imbalance classification from numpy import mean from sklearn.datasets import make_classification from sklearn.model_selection import GridSearchCV from sklearn.model_selection import RepeatedStratifiedKFold from sklearn.neighbors import KNeighborsClassifier from sklearn.calibration import CalibratedClassifierCV # generate dataset X, y = make_classification(n_samples=10000, n_features=2, n_redundant=0, n_clusters_per_class=1, weights=[0.99], flip_y=0, random_state=4) # define model model = KNeighborsClassifier() # wrap the model calibrated = CalibratedClassifierCV(model) # define grid param_grid = dict(cv=[2,3,4], method=['sigmoid','isotonic']) # define evaluation procedure cv = RepeatedStratifiedKFold(n_splits=10, n_repeats=3, random_state=1) # define grid search grid = GridSearchCV(estimator=calibrated, param_grid=param_grid, n_jobs=-1, cv=cv, scoring='roc_auc') # execute the grid search grid_result = grid.fit(X, y) # report the best configuration print("Best: %f using %s" % (grid_result.best_score_, grid_result.best_params_)) # report all configurations means = grid_result.cv_results_['mean_test_score'] stds = grid_result.cv_results_['std_test_score'] params = grid_result.cv_results_['params'] for mean, stdev, param in zip(means, stds, params): print("%f (%f) with: %r" % (mean, stdev, param)) |
Running the example evaluates the KNN with a suite of different types of calibrated probabilities on the imbalanced classification dataset.
Note: Your results may vary given the stochastic nature of the algorithm or evaluation procedure, or differences in numerical precision. Consider running the example a few times and compare the average outcome.
In this case, we can see that the best result was achieved with a “cv” of 2 and an “isotonic” value for “method” achieving a mean ROC AUC of about 0.895, a lift from 0.864 achieved with no calibration.
1 2 3 4 5 6 7 |
Best: 0.895120 using {'cv': 2, 'method': 'isotonic'} 0.895084 (0.062358) with: {'cv': 2, 'method': 'sigmoid'} 0.895120 (0.062488) with: {'cv': 2, 'method': 'isotonic'} 0.885221 (0.061373) with: {'cv': 3, 'method': 'sigmoid'} 0.881924 (0.064351) with: {'cv': 3, 'method': 'isotonic'} 0.881865 (0.065708) with: {'cv': 4, 'method': 'sigmoid'} 0.875320 (0.067663) with: {'cv': 4, 'method': 'isotonic'} |
This provides a template that you can use to evaluate different probability calibration configurations on your own models.
Further Reading
This section provides more resources on the topic if you are looking to go deeper.
Tutorials
Papers
- Predicting Good Probabilities With Supervised Learning, 2005.
- Class Probability Estimates are Unreliable for Imbalanced Data (and How to Fix Them), 2012.
Books
- Learning from Imbalanced Data Sets, 2018.
- Imbalanced Learning: Foundations, Algorithms, and Applications, 2013.
- Applied Predictive Modeling, 2013.
APIs
- sklearn.calibration.CalibratedClassifierCV API.
- sklearn.svm.SVC API.
- sklearn.tree.DecisionTreeClassifier API.
- sklearn.neighbors.KNeighborsClassifier API.
- sklearn.model_selection.GridSearchCV API.
Articles
- Calibration (statistics), Wikipedia.
- Probabilistic classification, Wikipedia.
- Platt scaling, Wikipedia.
- Isotonic regression, Wikipedia.
Summary
In this tutorial, you discovered how to calibrate predicted probabilities for imbalanced classification.
Specifically, you learned:
- Calibrated probabilities are required to get the most out of models for imbalanced classification problems.
- How to calibrate predicted probabilities for nonlinear models like SVMs, decision trees, and KNN.
- How to grid search different probability calibration methods on datasets with a skewed class distribution.
Do you have any questions?
Ask your questions in the comments below and I will do my best to answer.
How would you calibrate probabilities across a group, where the probabilities of the group must sum to one?
For example you might have 20 rows. The first 12 rows are group 1, the last 8 are group 2. Summing the probabilities should add up to 2, but they don’t. How would you calibrate in this case?
Thanks
Probabilities are considered for each row (sample), not across rows.
Perhaps I don’t understand what you are trying to achieve?
Am I right in assuming that creating and utilising your own loss function on the training of a Python SKlearn ML model is not possible without getting seriously under the bonnet. This is not the caseforr Models built in Keras or tuning the hyper marameters of ML models ?.
If I am wrong do you have a post on this ?
Thanks
You might have to extend the class and change the learning algorithm.
How would you set up a prediction problem where you are trying to predict a binary outcome for a group, where the sum of probabilities in the group sum to 1.
For example, you are predicting the probabilities for one group which has 3 observations. You use standard ML methods and your probabilities are 0.6, 0.4 and 0.25, which together sum to 1.25. But you need these to be calibrated to sum to 1. How would you optimise your ML in this way, to account for groups?
Does that make sense?
Love the articles by the way.
Thanks
Use a neural net with a softmax activation across the group.
Hi Jason,
In general, do you think that training and validation set should be combined to train a new model after probability is calibrated and optimal thresholds are picked on validation set? If combined and train a new model, is it valid to just use the previous selected thresholds on validation set?
Thanks!
It really depends on your dataset (how much data you have) and the specifics of your project.
Using a separate dataset for calibration and thresholding is ideal, but not always practical.
That makes sense, how would you recommend doing calibration and thresholding if there is not enough data?
You could use the training data (ouch! no!) or the same validation set for each task (better), or a separate validate set for each task (best).
hi jason, does it make sense to do a gridsearch first on your model, then run a calibrated classifier? https://datascience.stackexchange.com/questions/77181/do-not-understand-my-calibration-curve-output-for-xgboost < — this is what i got, is it along the correct line?? i do not get the plot /output i get for my isotnic and platts. it is an imbalanced priblem i have
If you are selecting a model based on predicted probabilities, then calibrate as part of the model and grid search on the calibrated model.
If not, then drop calibration completely, it likely is not needed.
ok, say i have xgboost – i run a grid search on this. i then get the parameters, i then run a fitted calibration on it: clf_isotonic = CalibratedClassifierCV(clf, cv=’prefit’, method=’isotonic’). my results are very strange for platts – i get only one point plotted for platts (between bin 0 and 0.2) do you think this is indicative of am imbalnced problem or should i not bother. for my uncalibrated plot, the curve is always underneath the diagonal (peffect calibration), but i do not understand why for platts i get only one point plotted
Perhaps platts is not appropriate for your model/dataset.
Is calibrating not preserve original order of the algorithm?
If it does then why would ROC AUC metric improve after we apply calibration?
i.e. ROC AUC depends on the order and if we do some monotonic transformation of probabilities that preserve original order it should not change, no?
https://stackoverflow.com/questions/54318912/does-calibration-improve-roc-score
Calibration wraps the original model.
ROC can improve because the probability distribution has changed which in turns changes the mapping to class labels via different thresholds as calculated in the ROC.
Ok, would it be then fair to say that if you had validation set in your example, AUC score on validation set in case of calibrated algorithm (trained and calibrated on train set) would not improve AUC score of uncalibrated algorithm on the same validation set which was trained as well on train set?
Not necessarily – it’s a change in scope.
I guess that is similar to comment to Abhilash Menon comment here (https://machinelearningmastery.com/calibrated-classification-model-in-scikit-learn/), can’t link to exact comment and replies from Pepe and John.
From there it sounds that order in calibrated set can be slightly different but that is simply averaging and CV artifacts. Otherwise, AUC and order should be exactly the same.
Then I don’t understand why in this article the AUC score improves that much. Can you please explain it?
Thanks for the tutorial Jason – this was a great read. Should calibration be performed before hyperparameter tuning? My understanding is that probability calibration should be performed first (especially when optimizing for ROC AUC or PR AUC, both of which use probabilities) followed by hyperparameter tuning on the calibrated model and model evaluation. Is this the general workflow? I’m also curious why oversampling/undersampling techniques would not be required (I assume this would be more dependent on the scoring metric you are interested in using for evaluation).
Lastly, is there any merit to not specifying the class weight argument for certain models in conjunction with probability calibration (not adjusting the margin to favor the minority class). For example, XGBoost’s scale_pos_weight argument gives greater weight to the positive class – I have read that disabling scale_pos_weight may give better calibrated probabilities (https://discuss.xgboost.ai/t/how-does-scale-pos-weight-affect-probabilities/1790).
Hard question.
Perhaps a better approach would be to incldues calibration as part of the “model” that is being tuned. This would be my preferred approach of the cuff.
Over/undersampling can help, it depends on your dataset. Try it and see.
Class weight and calibration may be useful if used together, I would guess not – but it’s hard to think through all possible cases. Again, perhaps try it and see.
Hello there, although it’s late but worth to mention it.
Actually it was my question and doubt too and I finally find an article that kind of answered that and give me clarification about all the “calibration” and also the “threshold moving” method.
So I definitely recommend that to every one else who has doubt and question in this fields and hope it helps others too. Following is the article link :
https://towardsdatascience.com/tackling-imbalanced-data-with-predicted-probabilities-3293602f0f2
Thank you for this article. I just started learning about dealing with imbalanced sets. I would like to understand why in all the examples above you choose to compare AUC. In your article about ROC AUC you say:
‘ROC curves should be used when there are roughly equal numbers of observations for each class.’
Wouldn’t it be better to look at PR AUC before and after calibration instead?
You’re welcome.
The choice of metric in this tutorial was arbitrary as we are focusing on calibration.
This can help you choose a metric:
https://machinelearningmastery.com/tour-of-evaluation-metrics-for-imbalanced-classification/
Dear Jason,
Thank you very much!
You’re welcome.
Hello Jason,
Thanks for writing up this excellent resource. I had a quick question. If I wanted to make something like a calibration plot (e.g., probability quantiles vs. label quantiles) to examine the calibrated model visually, would I still need to first separate the features and labels into a test/train, or does the k-folds cross-validation happening under the CalibratedClassifierCV mitigate that concern? i.e., can I use the same whole dataset for calibrating the model and then plotting the calibration curves with said model? Many thanks for your time.
If you’re using k-fold CV, the separation of train/test is done automatically. That’s what “cross-validation” means.
Hi Adrian,
Thanks for the reply. I understand what cross-validation does. I’m curious if we can use the same dataset that was used to calibrate the model (using CV) to generate calibration curves and get a sense of how well the model might adequately match the probabilities of future data. Or do I need yet another holdout set, separate from the data used during CV, to get a sense of how the calibrated model would perform on future data?
You can have another holdout set. If you do so, keep your holdout and pass the rest to cross-validation model. Then you the holdout set after your model is finished. This wikipedia post may be useful to you: https://en.wikipedia.org/wiki/Training,_validation,_and_test_sets
Many thanks, Adrian! This helps clear things up.
Thanks Jason for the great read! Are calibration plots less useful for highly imbalanced datasets? Whenever I have attempted calibrating model probabilities for models trained on highly imbalanced datasets the associated calibration plots show calibrated models being almost horizontal with the x-axis of the plot and nowhere near hugging the diagonal of what would represent a perfectly calibrated model (y-axis representing fraction of positive class and x-axis representing mean predicted probabilities of positive class). Is this more of a function of the severe class imbalance in the data or poorly calibrated model probability scores? Furthermore, if calibration plots are not useful for highly imbalanced datasets would you suggest using metrics like logloss and brier scores for evaluating how well model probabilities represent ‘true probabilities’ or are there other metrics and/or visuals you would suggest for evaluating this?
Hi Jake…You are very welcome! The following resource may be of interest to you:
https://machinelearningmastery.com/calibrated-classification-model-in-scikit-learn/
Hi Jason,
The det_curve return one value for each, theshold, fpr and fnr for my binary classification model(SVC). Can you please explain why does this happen.
Though there are score values in range 0-1
I agree with previous commenters that ROC/AUC might not be the best example to use in combination with calibration methods. See, for instance, stackoverflow. com/questions/54318912/does-calibration-improve-roc-score
Other than that, thanks for the great resource, as always!
Thank you rflameiro for your feedback and for sharing your findings!