Generative Adversarial Networks With Python Crash Course.
Bring Generative Adversarial Networks to Your Project in 7 Days.
Generative Adversarial Networks, or GANs for short, are a deep learning technique for training generative models.
The study and application of GANs are only a few years old, yet the results achieved have been nothing short of remarkable. Because the field is so young, it can be challenging to know how to get started, what to focus on, and how to best use the available techniques.
In this crash course, you will discover how you can get started and confidently develop deep learning Generative Adversarial Networks using Python in seven days.
Note: This is a big and important post. You might want to bookmark it.
Let’s get started.
- Update Jul/2019: Changed order of LeakyReLU and BatchNorm layers (thanks Chee).
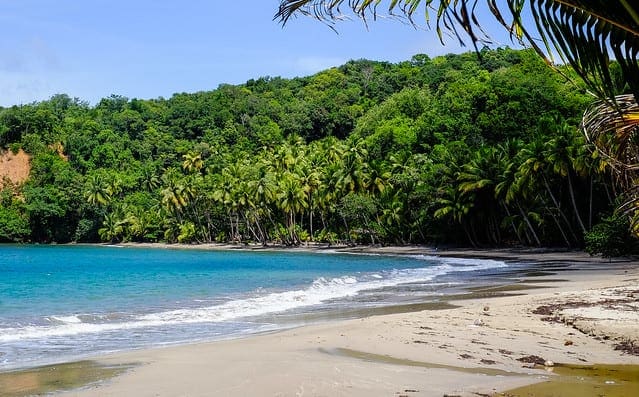
How to Get Started With Generative Adversarial Networks (7-Day Mini-Course)
Photo by Matthias Ripp, some rights reserved.
Who Is This Crash-Course For?
Before we get started, let’s make sure you are in the right place.
The list below provides some general guidelines as to who this course was designed for.
Don’t panic if you don’t match these points exactly; you might just need to brush up in one area or another to keep up.
You need to know:
- Your way around basic Python, NumPy, and Keras for deep learning.
You do NOT need to be:
- A math wiz!
- A deep learning expert!
- A computer vision researcher!
This crash course will take you from a developer that knows a little machine learning to a developer who can bring GANs to your own computer vision project.
Note: This crash course assumes you have a working Python 2 or 3 SciPy environment with at least NumPy, Pandas, scikit-learn, and Keras 2 installed. If you need help with your environment, you can follow the step-by-step tutorial here:
Crash-Course Overview
This crash course is broken down into seven lessons.
You could complete one lesson per day (recommended) or complete all of the lessons in one day (hardcore). It really depends on the time you have available and your level of enthusiasm.
Below are the seven lessons that will get you started and productive with Generative Adversarial Networks in Python:
- Lesson 01: What Are Generative Adversarial Networks?
- Lesson 02: GAN Tips, Tricks and Hacks
- Lesson 03: Discriminator and Generator Models
- Lesson 04: GAN Loss Functions
- Lesson 05: GAN Training Algorithm
- Lesson 06: GANs for Image Translation
- Lesson 07: Advanced GANs
Each lesson could take you anywhere from 60 seconds up to 30 minutes. Take your time and complete the lessons at your own pace. Ask questions and even post results in the comments below.
The lessons might expect you to go off and find out how to do things. I will give you hints, but part of the point of each lesson is to force you to learn where to go to look for help on and about deep learning and GANs (hint: I have all of the answers on this blog; just use the search box).
Post your results in the comments; I’ll cheer you on!
Hang in there; don’t give up.
Note: This is just a crash course. For a lot more detail and fleshed out tutorials, see my book on the topic titled “Generative Adversarial Networks with Python.”
Want to Develop GANs from Scratch?
Take my free 7-day email crash course now (with sample code).
Click to sign-up and also get a free PDF Ebook version of the course.
Lesson 01: What Are Generative Adversarial Networks?
In this lesson, you will discover what GANs are and the basic model architecture.
Generative Adversarial Networks, or GANs for short, are an approach to generative modeling using deep learning methods, such as convolutional neural networks.
GANs are a clever way of training a generative model by framing the problem as a supervised learning problem with two sub-models: the generator model that we train to generate new examples, and the discriminator model that tries to classify examples as either real (from the domain) or fake (generated).
- Generator. Model that is used to generate new plausible examples from the problem domain.
- Discriminator. Model that is used to classify examples as real (from the domain) or fake (generated).
The two models are trained together in a zero-sum game, adversarial, until the discriminator model is fooled about half the time, meaning the generator model is generating plausible examples.
The Generator
The generator model takes a fixed-length random vector as input and generates an image in the domain.
The vector is drawn randomly from a Gaussian distribution (called the latent space), and the vector is used to seed the generative process.
After training, the generator model is kept and used to generate new samples.
The Discriminator
The discriminator model takes an example from the domain as input (real or generated) and predicts a binary class label of real or fake (generated).
The real example comes from the training dataset. The generated examples are output by the generator model.
The discriminator is a normal (and well understood) classification model.
After the training process, the discriminator model is discarded as we are interested in the generator.
GAN Training
The two models, the generator and discriminator, are trained together.
A single training cycle involves first selecting a batch of real images from the problem domain. A batch of latent points is generated and fed to the generator model to synthesize a batch of images.
The discriminator is then updated using the batch of real and generated images, minimizing binary cross-entropy loss used in any binary classification problem.
The generator is then updated via the discriminator model. This means that generated images are presented to the discriminator as though they are real (not generated) and the error is propagated back through the generator model. This has the effect of updating the generator model toward generating images that are more likely to fool the discriminator.
This process is then repeated for a given number of training iterations.
Your Task
Your task in this lesson is to list three possible applications for Generative Adversarial Networks. You may get ideas from looking at recently published research papers.
Post your findings in the comments below. I would love to see what you discover.
In the next lesson, you will discover tips and tricks for the successful training of GAN models.
Lesson 02: GAN Tips, Tricks, and Hacks
In this lesson, you will discover the tips, tricks, and hacks that you need to know to successfully train GAN models.
Generative Adversarial Networks are challenging to train.
This is because the architecture involves both a generator and a discriminator model that compete in a zero-sum game. Improvements to one model come at the cost of a degrading of performance in the other model. The result is a very unstable training process that can often lead to failure, e.g. a generator that generates the same image all the time or generates nonsense.
As such, there are a number of heuristics or best practices (called “GAN hacks“) that can be used when configuring and training your GAN models.
Perhaps one of the most important steps forward in the design and training of stable GAN models is the approach that became known as the Deep Convolutional GAN, or DCGAN.
This architecture involves seven best practices to consider when implementing your GAN model:
- Downsample Using Strided Convolutions (e.g. don’t use pooling layers).
- Upsample Using Strided Convolutions (e.g. use the transpose convolutional layer).
- Use LeakyReLU (e.g. don’t use the standard ReLU).
- Use Batch Normalization (e.g. standardize layer outputs after the activation).
- Use Gaussian Weight Initialization (e.g. a mean of 0.0 and stdev of 0.02).
- Use Adam Stochastic Gradient Descent (e.g. learning rate of 0.0002 and beta1 of 0.5).
- Scale Images to the Range [-1,1] (e.g. use tanh in the output of the generator).
These heuristics have been hard won by practitioners testing and evaluating hundreds or thousands of combinations of configuration operations on a range of problems.
Your Task
Your task in this lesson is to list three additional GAN tips or hacks that can be used during training.
Post your findings in the comments below. I would love to see what you discover.
In the next lesson, you will discover how to implement simple discriminator and generator models.
Lesson 03: Discriminator and Generator Models
In this lesson, you will discover how to implement a simple discriminator and generator model using the Keras deep learning library.
We will assume the images in our domain are 28×28 pixels in size and color, meaning they have three color channels.
Discriminator Model
The discriminator model accepts an image with the with size 28x28x3 pixels and must classify it as real (1) or fake (0) via the sigmoid activation function.
Our model has two convolutional layers with 64 filters each and uses same padding. Each convolutional layer will downsample the input using a 2×2 stride, which is a best practice for GANs, instead of using a pooling layer.
Also following best practice, the convolutional layers are followed by a LeakyReLU activation with a slope of 0.2 and a batch normalization layer.
1 2 3 4 5 6 7 8 9 10 11 12 13 14 |
... # define the discriminator model model = Sequential() # downsample to 14x14 model.add(Conv2D(64, (3,3), strides=(2, 2), padding='same', input_shape=(28,28,3))) model.add(BatchNormalization()) model.add(LeakyReLU(alpha=0.2)) # downsample to 7x7 model.add(Conv2D(64, (3,3), strides=(2, 2), padding='same')) model.add(BatchNormalization()) model.add(LeakyReLU(alpha=0.2)) # classify model.add(Flatten()) model.add(Dense(1, activation='sigmoid')) |
Generator Model
The generator model takes a 100-dimensional point in the latent space as input and generates a 28x28x3.
The point in latent space is a vector of Gaussian random numbers. This is projected using a Dense layer to the basis of 64 tiny 7×7 images.
The small images are then upsampled twice using two transpose convolutional layers with a 2×2 stride and followed by a BatchNormalization and LeakyReLU layers, which are a best practice for GANs.
The output is a three channel image with pixel values in the range [-1,1] via the tanh activation function.
1 2 3 4 5 6 7 8 9 10 11 12 13 14 15 16 17 18 |
... # define the generator model model = Sequential() # foundation for 7x7 image n_nodes = 64 * 7 * 7 model.add(Dense(n_nodes, input_dim=100)) model.add(BatchNormalization()) model.add(LeakyReLU(alpha=0.2)) model.add(Reshape((7, 7, 64))) # upsample to 14x14 model.add(Conv2DTranspose(64, (3,3), strides=(2,2), padding='same')) model.add(BatchNormalization()) model.add(LeakyReLU(alpha=0.2)) # upsample to 28x28 model.add(Conv2DTranspose(64, (3,3), strides=(2,2), padding='same')) model.add(BatchNormalization()) model.add(LeakyReLU(alpha=0.2)) model.add(Conv2D(3, (3,3), activation='tanh', padding='same')) |
Your Task
Your task in this lesson is to implement both the discriminator models and summarize their structure.
For bonus points, update the models to support an image with the size 64×64 pixels.
Post your findings in the comments below. I would love to see what you discover.
In the next lesson, you will discover how to configure the loss functions for training the GAN models.
Lesson 04: GAN Loss Functions
In this lesson, you will discover how to configure the loss functions used for training the GAN model weights.
Discriminator Loss
The discriminator model is optimized to maximize the probability of correctly identifying real images from the dataset and fake or synthetic images output by the generator.
This can be implemented as a binary classification problem where the discriminator outputs a probability for a given image between 0 and 1 for fake and real respectively.
The model can then be trained on batches of real and fake images directly and minimize the negative log likelihood, most commonly implemented as the binary cross-entropy loss function.
As is the best practice, the model can be optimized using the Adam version of stochastic gradient descent with a small learning rate and conservative momentum.
1 2 3 |
... # compile model model.compile(loss='binary_crossentropy', optimizer=Adam(lr=0.0002, beta_1=0.5)) |
Generator Loss
The generator is not updated directly and there is no loss for this model.
Instead, the discriminator is used to provide a learned or indirect loss function for the generator.
This is achieved by creating a composite model where the generator outputs an image that feeds directly into the discriminator for classification.
The composite model can then be trained by providing random points in latent space as input and indicating to the discriminator that the generated images are, in fact, real. This has the effect of updating the weights of the generator to output images that are more likely to be classified as real by the discriminator.
Importantly, the discriminator weights are not updated during this process and are marked as not trainable.
The composite model uses the same categorical cross entropy loss as the standalone discriminator model and the same Adam version of stochastic gradient descent to perform the optimization.
1 2 3 4 5 6 7 8 9 10 11 12 13 14 |
# create the composite model for training the generator generator = ... discriminator = ... ... # make weights in the discriminator not trainable d_model.trainable = False # connect them model = Sequential() # add generator model.add(generator) # add the discriminator model.add(discriminator) # compile model model.compile(loss='binary_crossentropy', optimizer=Adam(lr=0.0002, beta_1=0.5)) |
Your Task
Your task in this lesson is to research and summarize three additional types of loss function that can be used to train the GAN models.
Post your findings in the comments below. I would love to see what you discover.
In the next lesson, you will discover the training algorithm used to update the model weights for the GAN.
Lesson 05: GAN Training Algorithm
In this lesson, you will discover the GAN training algorithm.
Defining the GAN models is the hard part. The GAN training algorithm is relatively straightforward.
One cycle of the algorithm involves first selecting a batch of real images and using the current generator model to generate a batch of fake images. You can develop small functions to perform these two operations.
These real and fake images are then used to update the discriminator model directly via a call to the train_on_batch() Keras function.
Next, points in latent space can be generated as input for the composite generator-discriminator model and labels of “real” (class=1) can be provided to update the weights of the generator model.
The training process is then repeated thousands of times.
The generator model can be saved periodically and later loaded to check the quality of the generated images.
The example below demonstrates the GAN training algorithm.
1 2 3 4 5 6 7 8 9 10 11 12 13 14 15 16 17 18 19 20 21 22 |
... # gan training algorithm discriminator = ... generator = ... gan_model = ... n_batch = 16 latent_dim = 100 for i in range(10000) # get randomly selected 'real' samples X_real, y_real = select_real_samples(dataset, n_batch) # generate 'fake' examples X_fake, y_fake = generate_fake_samples(generator, latent_dim, n_batch) # create training set for the discriminator X, y = vstack((X_real, X_fake)), vstack((y_real, y_fake)) # update discriminator model weights d_loss = discriminator.train_on_batch(X, y) # prepare points in latent space as input for the generator X_gan = generate_latent_points(latent_dim, n_batch) # create inverted labels for the fake samples y_gan = ones((n_batch, 1)) # update the generator via the discriminator's error g_loss = gan_model.train_on_batch(X_gan, y_gan) |
Your Task
Your task in this lesson is to tie together the elements from this and the prior lessons and train a GAN on a small image dataset such as MNIST or CIFAR-10.
Post your findings in the comments below. I would love to see what you discover.
In the next lesson, you will discover the application of GANs for image translation.
Lesson 06: GANs for Image Translation
In this lesson, you will discover GANs used for image translation.
Image-to-image translation is the controlled conversion of a given source image to a target image. An example might be the conversion of black and white photographs to color photographs.
Image-to-image translation is a challenging problem and often requires specialized models and loss functions for a given translation task or dataset.
GANs can be trained to perform image-to-image translation and two examples include the Pix2Pix and the CycleGAN.
Pix2Pix
The Pix2Pix GAN is a general approach for image-to-image translation.
The model is trained on a dataset of paired examples, where each pair involves an example of the image before and after the desired translation.
The Pix2Pix model is based on the conditional generative adversarial network, where a target image is generated, conditional on a given input image.
The discriminator model is given an input image and a real or generated paired image and must determine whether the paired image is real or fake.
The generator model is provided with a given image as input and generates a translated version of the image. The generator model is trained to both fool the discriminator model and to minimize the loss between the generated image and the expected target image.
More sophisticated deep convolutional neural network models are used in the Pix2Pix. Specifically, a U-Net model is used for the generator model and a PatchGAN is used for the discriminator model.
The loss for the generator is comprised of a composite of both the adversarial loss of a normal GAN model and the L1 loss between the generated and expected translated image.
CycleGAN
A limitation of the Pix2Pix model is that it requires a dataset of paired examples before and after the desired translation.
There are many image-to-image translation tasks where we may not have examples of the translation, such as translating photos of zebra to horses. There are other image translation tasks where such paired examples do not exist, such as translating art of landscapes to photographs.
The CycleGAN is a technique that involves the automatic training of image-to-image translation models without paired examples. The models are trained in an unsupervised manner using a collection of images from the source and target domain that do not need to be related in any way.
The CycleGAN is an extension of the GAN architecture that involves the simultaneous training of two generator models and two discriminator models.
One generator takes images from the first domain as input and outputs images for the second domain, and the other generator takes images from the second domain as input and generates images from the first domain. Discriminator models are then used to determine how plausible the generated images are and update the generator models accordingly.
The CycleGAN uses an additional extension to the architecture called cycle consistency. This is the idea that an image output by the first generator could be used as input to the second generator and the output of the second generator should match the original image. The reverse is also true: that an output from the second generator can be fed as input to the first generator and the result should match the input to the second generator.
Your Task
Your task in this lesson is to list five examples of image-to-image translation you might like to explore with GAN models.
Post your findings in the comments below. I would love to see what you discover.
In the next lesson, you will discover some of the recent advancements in GAN models.
Lesson 07: Advanced GANs
In this lesson, you will discover some of the more advanced GAN that are demonstrating remarkable results.
BigGAN
The BigGAN is an approach to pull together a suite of recent best practices in training GANs and scaling up the batch size and number of model parameters.
As its name suggests, the BigGAN is focused on scaling up the GAN models. This includes GAN models with:
- More model parameters (e.g. many more feature maps).
- Larger Batch Sizes (e.g. hundreds or thousands of images).
- Architectural changes (e.g. self-attention modules).
The resulting BigGAN generator model is capable of generating high-quality 256×256 and 512×512 images across a wide range of image classes.
Progressive Growing GAN
Progressive Growing GAN is an extension to the GAN training process that allows for the stable training of generator models that can output large high-quality images.
It involves starting with a very small image and incrementally adding blocks of layers that increase the output size of the generator model and the input size of the discriminator model until the desired image size is achieved.
Perhaps the most impressive accomplishment of the Progressive Growing GAN is the generation of large 1024×1024 pixel photorealistic generated faces.
StyleGAN
The Style Generative Adversarial Network, or StyleGAN for short, is an extension to the GAN architecture that proposes large changes to the generator model.
This includes the use of a mapping network to map points in latent space to an intermediate latent space, the use of the intermediate latent space to control style at each point in the generator model, and the introduction to noise as a source of variation at each point in the generator model.
The resulting model is capable not only of generating impressively photorealistic high-quality photos of faces, but also offers control over the style of the generated image at different levels of detail through varying the style vectors and noise.
For example, blocks of layers in the synthesis network at lower resolutions control high-level styles such as pose and hairstyle, blocks at higher resolutions control color schemes and very fine details like freckles and placement of hair strands.
Your Task
Your task in this lesson is to list 3 examples of how you might use models capable of generating large photorealistic images.
Post your findings in the comments below. I would love to see what you discover.
This was the final lesson.
The End!
(Look How Far You Have Come)
You made it. Well done!
Take a moment and look back at how far you have come.
You discovered:
- GANs are a deep learning technique for training generative models capable of synthesizing high-quality images.
- Training GANs is inherently unstable and prone to failures, which can be overcome by adopting best practice heuristics in the design, configuration, and training of GAN models.
- Generator and discriminator models used in the GAN architecture can be defined simply and directly in the Keras deep learning library.
- The discriminator model is trained like any other binary classification deep learning model.
- The generator model is trained via the discriminator model in a composite model architecture.
- GANs are capable of conditional image generation, such as image-to-image translation with paired and unpaired examples.
- Advancements in GANs, such as scaling up the models and progressively growing the models, allows for the generation of larger and higher-quality images.
Take the next step and check out my book on generative adversarial networks with python.
Summary
How Did You Do With The Mini-Course?
Did you enjoy this crash course?
Do you have any questions? Were there any sticking points?
Let me know. Leave a comment below.
There may be an error in the example code for the Discriminator and Generator model?
Shouldn’t the batch normalization layer be added before the activation?
i.e.
model.add(BatchNormalization())
model.add(LeakyReLU(alpha=0.2))
Possibly, it depends.
I’ll change it to meet convention. Thanks.
Hi Jason,
Thank you for the nice article.
Could you elaborate why the discrimator model should not be trainable?
I don’t understand how the generation could make sense if the discrimination is not optimized. I must miss something.
Cheers
Great question.
There is no loss function for the generator directly. Instead, we use the discriminator as the loss function and update the weights via the discriminator – e.g. the errors are propagated back through into the generator. When we do this, we only want to update the generator weights, not the discriminator as we can update the discriminator directly.
I explain more here:
https://machinelearningmastery.com/how-to-develop-a-generative-adversarial-network-for-a-1-dimensional-function-from-scratch-in-keras/
Hey, does it matter whether I use Keras or tf.keras?
All examples were developed using Keras, you can try using tf.keras, but I cannot confirm that it will work as described.
1.Two-Time-Scale Update Rule is proposed to provide individual learning rates for both generator and discriminator, because of convergence of GAN has not still been approved.
2.Frechet Inceptance Distance which find more similarities of generated images to real ones, rather than Inception Score.
3.GAN can learn more complex generative models for which maximum likelihood are not feasible.
4.Critic-Actor learning has been analyzed using stochastic approximation indicating, that TTUR ensures that GAN training reaches a stationary local Nash equilibrium if critic learns faster than actor, then Convergence is proved via Ordinay Differential Equation whose stable limit points coincide with stationary local Nash equilibrium.
5.In new GAN, discriminator learns faster rather than generator, due to avoiding overfitting in current discriminator
Well done!
Dear sir,
Thank you for sharing your knowledge
Could you kindly tell me how to load my dataset if it is csv file?
See this tutorial on how to load data from CSV:
https://machinelearningmastery.com/load-machine-learning-data-python/
Hi Jason,
For the task of Day 1:
Finer applications of GAN, I’ve found are:
1.Text to image translation;
2.Improvising the models that have poor training datasets through GAN by giving increased training samples by data augmentation;
3.Generating Different poses of human faces to train a bio metrics face recognition system eminent to identify a person even at adverse face postures.
Well done!
Day 2 task:
Few more GAN hacks:
1. Use an alternative loss function i.e., instead of min log D use max log D
2.sample from a Gaussian distribution instead of normal distribution
3.Addition of Gaussian noise to every layer of Generator
Great work!
For Day 1 on the tutorial, some possible GAN applications (I am focusing on text GAN):
1. machine translation: translate text or speech from one language to another
2. language modeling: one or more sequence of words that follow a sentence or part of a sentence and
3. text summarization: providing a high level summary of a large set of sentences or corpus
Well done!
For Day-1…
I tried to run before I can walk.
Purchased Probability for Machine learning in confirmation of my student status.
Am wondering if there exists a probability distribution of people biting-off more than they can chew in the field of AI and how that can be predicted from their history of accessing your Gentle Introductions and mini-courses.
Harikrishna’s focus matches mine.
I think we must bite off more than we can chew in order to grow.
Hang in there and contact me if I can help along the way.
https://machinelearningmastery.com/contact/
Day 3 bonus question:
# define the discriminator model
model = Sequential()
# downsample to 32×32
model.add(Conv2D(64, (3,3), strides=(2, 2), padding= ‘same’ , input_shape=(64,64,3)))
model.add(LeakyReLU(alpha=0.2))
model.add(BatchNormalization())
# downsample to 16×16
model.add(Conv2D(64, (3,3), strides=(2, 2), padding= ‘same’ ))
model.add(LeakyReLU(alpha=0.2))
model.add(BatchNormalization())
# classify
model.add(Flatten())
model.add(Dense(1, activation= ‘sigmoid’ ))
# define the generator model
model = Sequential()
# foundation for 7×7 image
n_nodes = 64 * 8 * 8
model.add(Dense(n_nodes, input_dim=100))
model.add(LeakyReLU(alpha=0.2))
model.add(BatchNormalization())
model.add(Reshape((8, 8, 64)))
# upsample to 16×16
model.add(Conv2DTranspose(64, (3,3), strides=(2,2), padding= ‘same’ ))
model.add(LeakyReLU(alpha=0.2))
model.add(BatchNormalization())
# upsample to 32×32
model.add(Conv2DTranspose(64, (3,3), strides=(2,2), padding= ‘same’ ))
model.add(LeakyReLU(alpha=0.2))
model.add(BatchNormalization())
#upsample to 64×64
model.add(Conv2DTranspose(64, (3,3), strides=(2,2), padding= ‘same’ ))
model.add(LeakyReLU(alpha=0.2))
model.add(BatchNormalization())
model.add(Conv2D(3, (3,3), activation= ‘tanh’ , padding= ‘same’ ))
Thanks Jason !
Well done!
Day 04 – GAN Loss Functions
Three additional types of loss function that can be used to train the GAN models:
Minimax loss
Wasserstein loss
Least squares loss
I am actually confused with respect to the Minimax loss – it seems to be the binary crossentropy loss that you defined. I thus have a couple questions:
1) You compile the Discriminator with binary_crossentropy and Adam, but then you say that the Discriminator does not need to be trained… am I missing something?
2) does GAN_model.compile(loss= loss_function , optimizer=optimizer) where the GAN_model is built sequentially as in the lesson intrinsically effectuate the minimax adversarial game ?
Thanks so much Jason!
Good question, this will clarify things:
https://machinelearningmastery.com/how-to-code-the-generative-adversarial-network-training-algorithm-and-loss-functions/
Ah ! So when you calculate the discriminator loss, it is certainly trainable but when you train the GAN, the discriminator is not trained. Is that right ?
No, the discriminator and the generator are both trained.
Day 5: GAN Training Algorithm
import tensorflow as tf
from tensorflow.keras import datasets, layers, models
from tensorflow.keras.models import Sequential
from tensorflow.keras.layers import Conv2D, LeakyReLU, BatchNormalization, Flatten, Dense, Reshape, Conv2DTranspose
from tensorflow.keras.optimizers import Adam
import matplotlib.pyplot as plt
from sklearn.model_selection import train_test_split
import numpy as np
# define the discriminator model
model = Sequential()
# downsample to 14×14
model.add(Conv2D(64, (3,3), strides=(2, 2), padding= ‘same’ , input_shape=(28,28,1)))
model.add(LeakyReLU(alpha=0.2))
model.add(BatchNormalization())
# downsample to 7×7
model.add(Conv2D(64, (3,3), strides=(2, 2), padding= ‘same’ ))
model.add(LeakyReLU(alpha=0.2))
model.add(BatchNormalization())
# classify
model.add(Flatten())
model.add(Dense(1, activation= ‘sigmoid’ ))
# define the generator model
gmodel = Sequential()
# foundation for 7×7 image
n_nodes = 64 * 7 * 7
gmodel.add(Dense(n_nodes, input_dim=100))
gmodel.add(LeakyReLU(alpha=0.2))
gmodel.add(BatchNormalization())
gmodel.add(Reshape((7, 7, 64)))
# upsample to 14×14
gmodel.add(Conv2DTranspose(64, (3,3), strides=(2,2), padding= ‘same’ ))
gmodel.add(LeakyReLU(alpha=0.2))
gmodel.add(BatchNormalization())
# upsample to 28×28
gmodel.add(Conv2DTranspose(64, (3,3), strides=(2,2), padding= ‘same’ ))
gmodel.add(LeakyReLU(alpha=0.2))
gmodel.add(BatchNormalization())
gmodel.add(Conv2D(1, (3,3), activation= ‘tanh’ , padding= ‘same’ ))
generator = gmodel
discriminator=model
discriminator.compile(loss=’binary_crossentropy’, optimizer=Adam(lr=0.0002, beta_1=0.5))
discriminator.trainable=False
GAN=Sequential()
GAN.add(generator)
GAN.add(discriminator)
GAN.compile(loss=’binary_crossentropy’, optimizer=Adam(lr=0.0002, beta_1=0.5))
discriminator.trainable=True #because the d_loss needs it… am I wrong ?
n_batch = 16
latent_dim = 100
(train_images, train_labels), (_, _) = tf.keras.datasets.mnist.load_data()
train_images = train_images.reshape(train_images.shape[0], 28, 28, 1).astype(‘float32’)
train_images = (train_images – 127.5) / 127.5 # Normalize the images to [-1, 1]
def generate_fake_samples(generator, latent_dim, n_batch):
generated=generator.predict(tf.random.normal(shape=(n_batch,latent_dim)))
return generated
def generate_latent_points(latent_dim,n_batch):
return tf.random.normal(shape=(n_batch,latent_dim))
for i in range(10000):
X_real,_ = train_test_split(train_images, train_size=n_batch)
y_real = tf.ones(tf.constant([len(X_real)]))
X_fake=generate_fake_samples(generator, latent_dim, n_batch)
y_fake = tf.zeros(tf.constant([len(X_fake)]))
X, y = np.vstack((X_real, X_fake)), np.vstack((y_real, y_fake))
y=np.reshape(y,n_batch*2)
d_loss = discriminator.train_on_batch(X, y)
X_gan = generate_latent_points(latent_dim, n_batch)
y_gan = tf.ones((n_batch, 1))
g_loss = GAN.train_on_batch(X_gan, y_gan)
Thanks in advance if you could please answer the question in the code about discriminator.trainable=True/False !
Best
Well done!
Hi Jason,
Day 01 Task:
Before that I would like to ask, Does the model use some security algorithms too so that it should not be misuse these techniques for bad things(just being Realistic) as we are getting realistic fake data?
I found some Applications are as follows:
1) Image to Image Translation : We can use GAN model to generate images of galaxies and can study them
2) Voice Translation : Using GAN we can do audio style transfer such as Jazz to Classical music
3) We can generate images from scratch having summary of statistics for studying nature of dark matter or dark energy in the Cosmos
Well done!
Hi Jason,
Day 02 Task:
Some tips and hacks i found are as follows:
1) Image Data Augmentation : We can use this technique while training.As it is used to expand the training dataset in order to improve the performance of GAN model
2) One sided label smoothing : We can use this to avoid overconfidence and overfitting
3) Loss functions : for better performace of model in the training(source:WGAN paper)
4) Add noise to the real and generated data before feeding it to the Discriminator
5) Orthogonal Regularization(source: BigGAN)
Well done!
Hi Jason,
Task of Day 1:
Applications of GAN are
1.Dataset Augmentation which is widely used application.
2.Different types of translations like image to image and text to image translation.
3.Motion Stabilization & Super Resolution which can generate sharp images from a blur image.
Nice work!
Day 02 Task:
Some tips and hacks are as follows:
1) Loss Function plays an important role in training so proper loss function could be used while training. We can use Wasserstein loss function which is used in the WGAN paper.
2) Mode Collapse which is the pain area while training a GAN model and it can be solved with parameters like Learning Rate. With thi issue model will start generating the same modes of images and it will forget to generate other modes.
3) Soft and Noisy labels which is important while training the Discriminator. For example, fake image label would be between 0 to 0.1 and for ream it would be from 0.9 to 1.0
Well done!
Hi Jason,
Task of Day 1
1. Data Augmentation for Training and Testing applications
2. Augment images / Videos for personalization experience
3. Creative Art to enhance human creativity
Well done!
jo Jason,
Task of Day 2
Additional GAN Hacks and Tips during training
1. Use a Gaussian Latent Space – This is to ensure randomness while generating inputs
2. Separate Batches of Real and Fake Images to make sure that the Data are structurally clean and manageable
3. Use Noisy Labels – To mix real and fake data
Great work!
Day 3 Bonus Question
#DEscriminator , Generator Model Code to accept 64 X 64 X 3 Size input
# Descriminator Model Design
model = Sequential()
#Extract 64 Feature maps and down size the input to 50% 32 X 32
model.add(Conv2D(64, (3,3), strides = (2,2), padding=’same’, input_shape=(64,64,3)))
model.add(LeakyReLU())
model.add(BatchNormalization())
#Extract 64 Feature maps and down size the input to 50% 16 X 16
model.add(Conv2D(64, (3,3), strides = (2,2), padding=’same’))
model.add(LeakyReLU())
model.add(BatchNormalization())
#Classify the input
model.add(Flatten())
model.add(Dense(1, activation=’sigmoid’))
print(model.summary())
# Define Generator Model
model = Sequential()
#Foundation for Nodes
n_node = 64 * 16* 16
model.add(Dense(n_node,input_dim=100))
model.add(LeakyReLU())
model.add(BatchNormalization())
model.add(Reshape((16,16,64)))
#Upsample 32 X 32
model.add(Conv2DTranspose(64, (3,3), strides =(2,2), padding =’same’))
model.add(LeakyReLU(alpha=0.2))
model.add(BatchNormalization())
#UpSample 64 X 64
model.add(Conv2DTranspose(64, (3,3), strides=(2,2), padding = ‘same’))
model.add(LeakyReLU(alpha=0.2))
model.add(BatchNormalization())
model.add(Conv2D(3,(3,3),activation=’tanh’, padding=’same’))
print(model.summary())
Well done!!
Day 4 Task
Additional Types of Loss functions for GAN
1. acgan
2. Least Squares
3. minimax
4. wasserstein
When I saw the implementation in py for these losses it was always referred separately as xxx_generator_loss and xxx_discriminator loss so my question is when we specify the losses we do not specify this for generator or discriminator but the implementation of above losses are different. Unable to understand how does this works or is it that so far we have used only binary_crossentropy but for advanced implementation we need to specify
Nice work!
Some loss functions require a custom function, some can be used from the library directly. It depends.
Lesson 1:
First, I’ll attempt finding 3 applications for GANs then do some research and add it to my post.
1. Generating human-looking figures (eg for use in movies as digital “actors”)
2. Creating artwork to replicate a well-known artist’s work (but beware usage of such products to defraud)
3. Could they be used for textual reproductions that resemble a well-known author’s works?
Now for what my research suggests:
1. Photo editing to clean up photos
2. Face aging; this could be used in forensics and to track either missing people or criminals years after an incident
3. Virtual clothing try-outs
The first two of my pre-research suggestions were also in the research I found. The article did mention text to image, but not text- to-text, so not sure if my 3rd suggestion is valid.
Research source: https://machinelearningmastery.com/impressive-applications-of-generative-adversarial-networks/
Well done!
Great Tutorial Jason, Thank You ! I found more GAN hacks over and above what you have discussed in the following link
https://github.com/soumith/ganhacks
Thanks!
Nice tutorial. GAN can do marvelous staff in different applications, like: data augmentation in biomedical signals, image replication, textual correction.
Thanks!
Hi Jason,
Day 01 Task:
1- Generating image
Wang et al, Generative Image Modeling using Style and Structure Adversarial Networks.
Two-step generation :
– Sketch → Color.
– Binomial random seed instead of Gaussian.
2- Translating image
Perarnau et al, Invertible Conditional GANs for image editing.
3- Domain adaptation
Taigman et al, Unsupervised cross-domain image generation.
Well done!
Day 1 task:
1. Generating high quality test data (which can be a pain to produce)
2. Producing cartoon images from real photos
3. Generating audio in a particular style (accent/intonation) – I don’t know if this is actually possible!
For (3) – it is actually possible. This may be related: https://blog.tensorflow.org/2020/01/building-ai-empowered-music-library-tensorflow.html
Day 1 task:
First of all id like to thank you for offering this 7 day crash course for free. It will sure help me in my studies!
GAN’s can be used, as stated, in various tasks that relate to computer vision. Seeing as we are currently living through a pandemic, the applications I found and propose are:
1. Generating a set of high quality x ray images to use for training other neuronal networks, obtaining better results.
2. Once the discriminator is trained well enough, it could also be used to classify images
3. Supersampling an image. For example, grabbing a low quality image of an x ray per say, running it through a GAN, and generating a much higher quality version.
Thank you for the feedback Wilson! Keep up the great work!
Day 2 Task:
While researching and trying to train my own GAN I found these tips that were not mentioned in the lesson:
1. Track failures early. By printing the output of the loss of the discriminator and generator on each epoch we can realize if the training is going well or not, preventing us from wasting time training a model that is not correct.
2. Don balance loss via statistics. Most of the time its pointless to train the generator or discriminator more based on how their loss function is outputing. Might as well wait until its finished or update the inputs before trying again.
3. Modifying the learn rates. Ive had this work sometimes, not always. If we notice that the generator is unable to produce good copies that the discriminator is learning to quickly how to find fakes, and vise versa, if the generator loss function steadily decreases, then its fooling D with garbage.
Thank you for the feedback Wilson!
Hello dear sir,
After running the code I faced the followinge error:
“Unknown loss function: binary-crossentropy. Please ensure this object is passed to the ‘custom_objects’ argument.”
What should I do for solving that?
Thank you
Hi Pouya…Did you copy and past the code or type it in? Also, you may want to implement it also in Google Colab.
Actually I made some changes, I will try it in Google Colab. Thank you.
Thank you for the feedback! Keep up the great work!
GAN IS USED FOR IMAGE TO IMAGE TRANSATION
GAN FOR STEGANOGRAPHY
GAN FOR SPEECH REPRODUCTION
Thank you for the feedback Celia! You may find the following of interest:
https://machinelearningmastery.com/impressive-applications-of-generative-adversarial-networks/
I came upto cycle gan where I got stuck with an error I couldnot rectify even though i tried with what is given in stackoverflow Its about DS_store which is aMACOS hidden file Iam using anormal COLAB in HP i3 processor
Hi Sankar…What error messages are you receiving? Also, you may want to the GPU option in Google Colab.
Haii,
I just started to learn GAN and I read some articles and tutorials. It says that the generator is updated by the loss function. But I still didn’t understand how or from where the generator got information of real image to copy and generate similar image from a randomly generated noise.
Hi Fidha…You may want to start here:
https://machinelearningmastery.com/start-here/#gans
I’ve just done lesson one. This is really useful. You asked for three examples of applications for GANs. This is my thoughts:
1. Generating mood music, based on the mood that is required, e.g. for movies or in public spaces.
2. Producing summarised transcripts of conversations, e.g. in conference calls, that enable translation and/or assistance for people with hearing difficulties
3. Generating labels for training data in supervised training models
Great practical examples Nic! Let us know if you have any questions regarding the content of the mini-course.
Lesson 01: What Are Generative Adversarial Networks?
At our institution, we have over 5,000 doctoral dissertations in pdf format. I am thinking how I could apply GANs on a selected dataset from these PhD dissertations.
Thank you Dusan for your feedback! Let us know how your project goes!
Lesson 02: What Are Generative Adversarial Networks?
It is interesting that there are a large number of GAN methods on the Internet. Some of them are:
1. Transforming an image from one domain to another (CycleGAN),
2. Generating an image from a textual description (text-to-image),
3. Generating very high-resolution images (ProgressiveGAN) and many more.
Three applications of GAN:
1) Improve Cybersecurity
Hackers manipulate images by adding malicious data to them. GANs can be trained to identify such instances of fraud.
2) Improve Healthcare
GANs can assist is drug discovery. Researchers can train the generator with the existing database to find new compounds that can potentially be used to treat new diseases.
3)Text-to-image synthesis
GAN Networks can generate images conditioned on text descriptions.
Thank you for your feedback Rohit! Keep up the great work in the course!